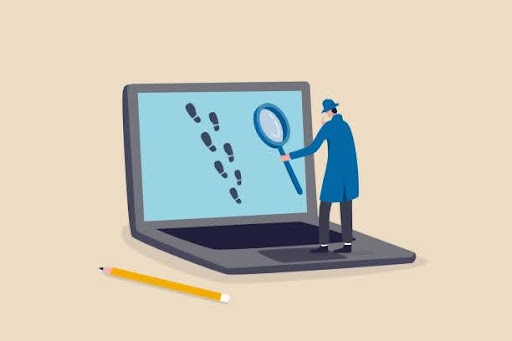
Modern worldwide connections produce digital traces from every activity we carry out online. Each visit to a website together with all our activities on purchases and social media constitute our digital footprint. Digital footprint analysis emerges as a key technology to analyze user behavior patterns because privacy concerns and security issues alongside personalization needs have intensified. The implementation of Artificial Intelligence (AI) and Machine Learning (ML) systems represents the main catalyst for this evolutionary trend.
Understanding Digital Footprints
The internet activity of every individual generates their digital footprint which leaves complete records of their online actions behind. The analytical study examines two types of data tracks: active footprints which include social media posts and emails as well as blog comments and passive footprints which incorporate IP addresses and anonymous search histories that manufacturers obtain without consent. The advantages of digital footprints through personalization and convenience come with risks that include privacy breaches and identity theft and digital profiling. An increasing quantity of individually produced data presently makes manual analysis impractical. AI together with machine learning starts its operation when human capability reaches its limit.
The Role of AI in Digital Footprint Analysis
Through its capabilities Artificial Intelligence drives systems to evaluate extensive data in shortened periods. Through the integration of natural language processing (NLP) and predictive analytics Artificial Intelligence systems examine and understand and extract conclusions from users' digital actions in real time. Algorithms powered by AI detect behavioral patterns through the analysis of online search activities and application usage as well as online purchasing behavior. The obtained data enables businesses to generate suitable recommendations while security systems benefit from detecting suspicious and dangerous digital activities. The capability of digital footprint checker grows more sophisticated because of AI implementation over time. The systems develop advanced abilities through new data interaction which leads to threat detection enhancements together with user experience customization abilities.
Machine Learning: Powering Prediction and Personalization
Machine learning, a subset of AI, plays a crucial role in predictive modeling. When trained with past data ML systems develop capabilities to forecast the way users will behave moving forward. Evaluation becomes possible through digital footprints to foresee the next purchase selection and voting patterns and potential medical concerns of customers.
Risk scoring along with fraud detection relies heavily on ML models for operation. The digital trail of an individual becomes the foundation for financial institutions to establish credit ratings and discover suspected cases of identity theft. Insurance organizations apply this data to analyze policyholder dangers. The hidden data correlations discovered by machine learning assist in generating relevant insights from digital user records that would be impossible for human analysts to uncover.
Ethical and Privacy Concerns
The implementation of AI and ML technologies for digital footprint analysis creates substantial ethical dilemmas for users. Who owns this data? How is it being used? Is the consent truly informed? The problem of data exploitation intensifies because people do not know when their data gets collected or when their behavior gets influenced. The distinction between helpful manipulation and deceptive tactics in political campaigns and individualized advertising is growing difficult for people to discern. Significant problems arise when AI models employ bias within their training data which generates discriminatory results particularly in human resources and credit evaluation and enforcement procedures. Companies promoting ethical data usage need to use transparent AI systems and establish data governance policies while following GDPR (General Data Protection Regulation) standards.
What the Future Holds
Technological advancement will lead to improved sophisticated methods of digital footprint analysis that incorporate AI and ML. Digital tools will advance into accurate and context-specific instruments. Digital users will significantly benefit from XAI technologies because these systems provide explanations about decisions that emerge from their digital information. Digital footprint monitoring systems gradually will develop into real-time monitoring capabilities while automated privacy audits alongside personal data dashboards for active user-managed online presence will emerge as future applications. The digital age will make digital footprint analysis a crucial part of personal branding practices as well as online reputation management.
Federated learning presents an opportunity to process data on devices instead of transfer it to central servers thus preserving privacy and enabling strong AI analysis capabilities.
Conclusion
Through this combination of AI and machine learning technology with digital footprint analysis the digital world experiences a transformation. The technology unlocks various personalization options while providing security tools and behavioral data insights however this must accompany absolute ethical conduct and strict protection for user privacy.
Future users and organizations must maintain equilibrium between innovation development and ethical responsibility to create digital experiences that protect privacy without compromising smartness and respect.